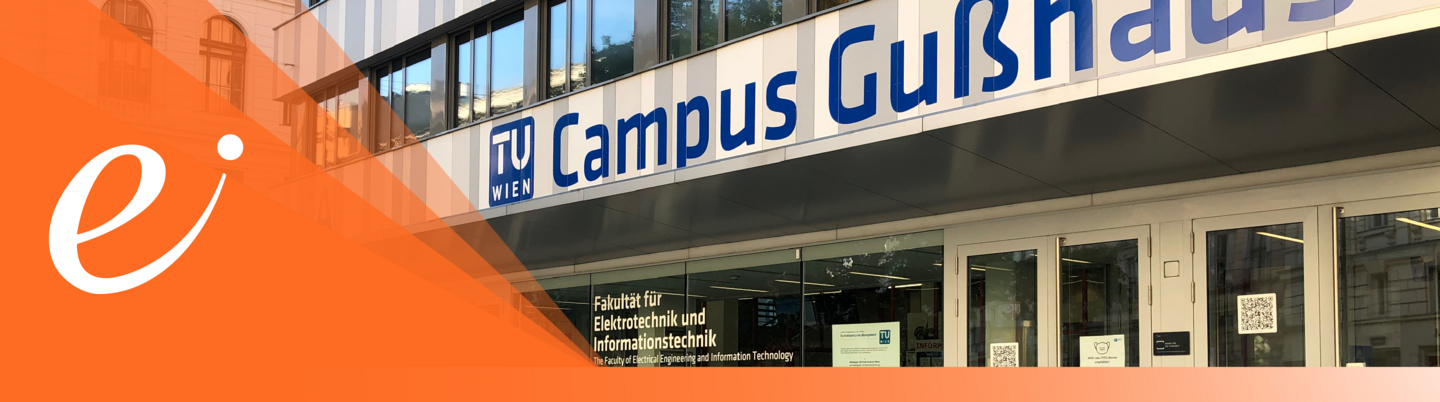
The ICT would like to congratulate Dipl.-Ing. Matthias Bittner for receiving the faculty award of the Faculty of Electrical Engineering and Information Technology for the presentation of his master thesis
Pattern Recognition in a heterogeneous Smart Grid environment
The ongoing increase of decentralized mostly renewable energy producers as well as the growth of electromobility pose big challenges for our future power grid. The Energy&IT group of ICT is actively developing new concepts and solutions and is therefore particularly proud of the successful Smart Grid research cooperation with Siemens AG Austria, which made this master thesis possible.
Matthias will also continue to work with us in the future as part of his PhD in the ICT’s CD Lab for Embedded Machine Learning, and we look forward to further exciting projects and a further strengthening of the collaboration with Siemens.
Abstract of the thesis: Considering a Smart Grid and just observing the sampled grid measurements is an old-fashioned and outdated way of looking at this highly dynamical and heterogeneous system. There is a strong need of involving the environmental (e.g., weather, seasonal behaviour) and heterogeneous (e.g., diverse energy sources and consumers) influences into their analysis and optimization. This thesis is therefore starting at a very abstract viewpoint of such a Smart Grid and proposes: a pipeline for extracting patterns and a design cycle for developing Machine Learning concepts. The pattern extraction pipeline provides methods and concepts for extracting patterns related to the environmental and heterogeneous influences. This step of revealing and extracting patterns is achieved by applying this pipeline on historical data of an existing testbed in Aspern Vienna, Austria. The second part of this thesis is then focused on proposing a Machine Learning design cycle, which provides a general methodology for developing Machine Learning concepts based on the extracted patterns. This results in concepts for power consumption forecasting based on environmental data, system state clustering and rare event detection. The overall aim of all these concepts is to optimize the functionality, reliability and efficiency of the modern Smart Grids.
Supervisors: Dipl.-Ing. Daniel Hauer, Ao.Univ.Prof. Dr. Thilo Sauter